The Art of Predictive Analytics: Forecasting Demand with Explanatory Variables
Leverage predictive analytics to forecast demand using explanatory variables. Uncover insights to optimize your business strategy and drive growth.
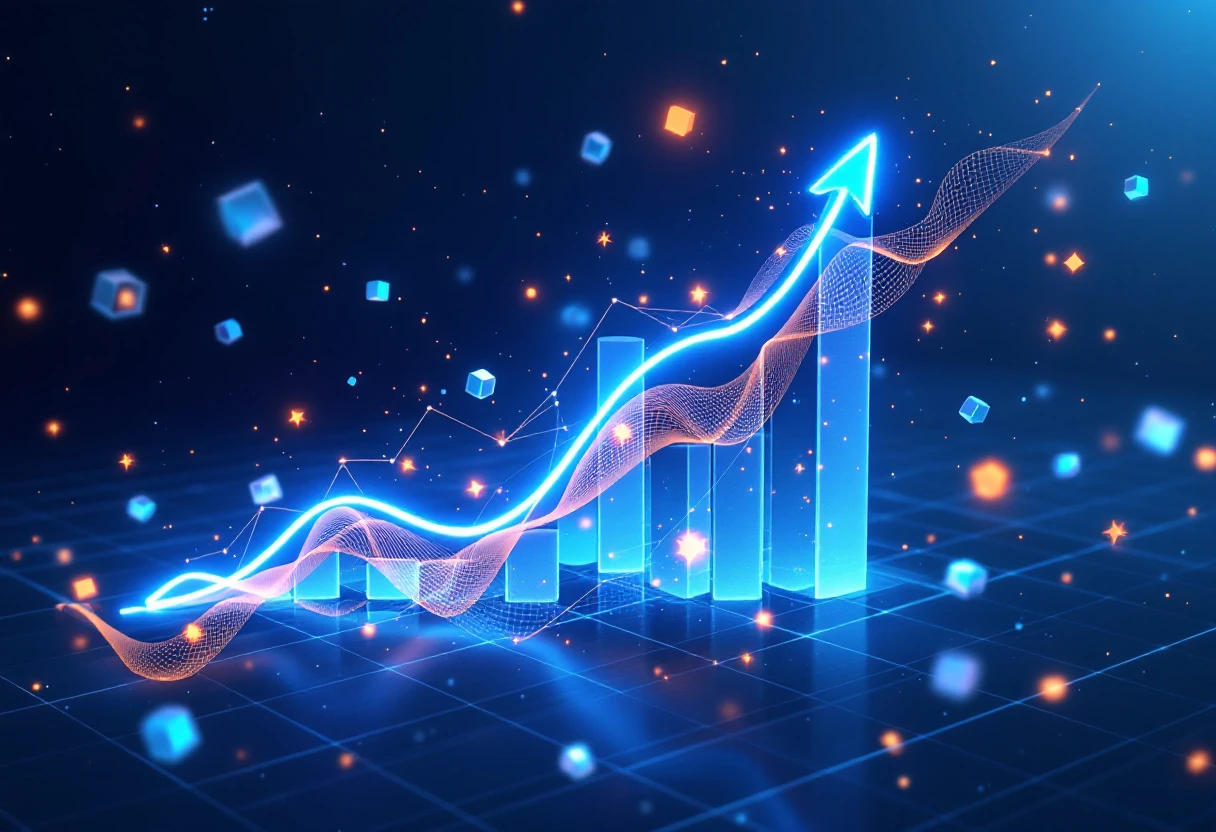
In today's data-driven business landscape, simply guessing what your customers might want next isn't going to cut it. That's where predictive analytics comes in - it's like having a crystal ball, but one that's powered by cold, hard data and scientific methods.
Understanding Explanatory Variables in Demand Forecasting
When we talk about forecasting demand, we're not just looking at historical sales data. The real magic happens when we factor in explanatory variables - those external and internal factors that influence buying behavior. As product analytics and inventory insights show, these variables can make or break your predictions.
Key Explanatory Variables to Consider:
- Seasonal Factors: Weather patterns, holidays, and annual events
- Economic Indicators: GDP, unemployment rates, consumer confidence
- Marketing Activities: Promotional campaigns, advertising spend, social media presence
- Competitive Landscape: Competitor pricing, new market entrants
- Internal Factors: Price changes, product availability, website traffic
Building a Robust Forecasting Model
The key to accurate demand forecasting isn't just collecting data - it's about making sense of it. Modern AI-powered tools can process multiple variables simultaneously, identifying patterns and correlations that human analysts might miss. The debate between human and machine capabilities in forecasting continues, but the truth is, the best results come from combining both.
Steps to Implement Predictive Analytics:
- Data Collection and Cleaning
- Variable Selection and Analysis
- Model Development and Testing
- Implementation and Monitoring
- Continuous Refinement
Breaking Down Silos for Better Predictions
One of the biggest challenges in implementing effective predictive analytics is the isolation of different departments. Breaking down silos between marketing and inventory teams is crucial for accurate forecasting. When teams share data and insights, the quality of predictions improves dramatically.
Handling Seasonal vs. Regular Demand
Different products require different forecasting approaches. Understanding the differences between seasonal and evergreen inventory planning is crucial for accurate predictions. Your model needs to account for these variations and adjust accordingly.
Real-World Applications and Benefits
When implemented correctly, predictive analytics with explanatory variables can:
- Reduce stockouts and overstock situations
- Optimize marketing spend based on predicted demand
- Improve customer satisfaction through better product availability
- Enable more efficient resource allocation
- Provide competitive advantages through data-driven decisions
Common Pitfalls to Avoid
While predictive analytics is powerful, there are several common mistakes to watch out for:
- Overlooking data quality issues
- Ignoring important variables
- Over-relying on historical data
- Not validating predictions regularly
- Failing to adjust models based on changing conditions
Looking to the Future
As technology evolves, predictive analytics is becoming more sophisticated and accessible. AI and machine learning are making it possible to process more variables and generate more accurate predictions than ever before. The key is staying adaptable and ready to embrace new methodologies as they emerge.
Remember, successful demand forecasting isn't about finding a one-size-fits-all solution. It's about developing a flexible, data-driven approach that can evolve with your business needs. By leveraging explanatory variables effectively, you can create more accurate predictions and make better business decisions.
The future of business planning lies in smart, data-driven decision-making. Whether you're managing inventory, planning marketing campaigns, or making strategic business decisions, understanding and implementing predictive analytics with explanatory variables is no longer optional - it's essential for staying competitive in today's market.